Machine Learning Roulette
Offer valid for first time depositors in 888casino only. Players making their first time 888casino Machine Learning Roulette deposit in this promotion will be excluded from the standard 888casino sign on bonus. One of the great things about machine learning is that there are so many different approaches to solving problems. Neural networks are great for finding patterns in data, resulting in predictive capabilities that are truly impressive. Reinforcement learning uses rewards-based concepts, improving over. The simplest roulette computer I offer is called the 'Basic roulette computer'. It is just a basic roulette computer using the basic design described above. It is FREE to my roulette system players because it realistically can beat only perhaps 5% of wheels, and still the accuracy is nowhere what could be achieved. Your system is a combination of believing the roulette wheel has a memory (the 'hot' and 'icy' numbers graphics in Roulette wheels in Vegas are meant to entice suckers with 'systems' that don't work). You also throw in the Martingale system - which goes like this. Make a bet - if you lose, make a larger bet that covers all your.
- Machine Learning Roulette App
- Video Roulette Machines In Casinos
- Machine Learning Roulette Sites
- Roulette Machines For Sale
Every now and then I need to implement a proportional selection function for a machine learning technique. I was thinking about the k-means++ clustering algorithm. It uses proportional selection.
A common algorithm to implement proportional selection is called roulette wheel selection. I hadn’t coded such a function in several months so I figured I’d do so to keep in practice.
My example set up an array of 5 values:
The idea of proportional selection is to randomly pick one of the values (well the index of the value) in a way that is proportional to the magnitude of the values. So any of the 5 items could be selected, but item [3] should have the largest probability of being selected.
For my demo data, the sum of the 5 values is 20 + 50 + 30 + 60 + 40 = 200. So the probability of each item being selected is the value divided by the sum:
The next step is to compute the cumulative probabilities:
Notice the cumulative probabilities array has an extra cell at the beginning with 0.0.
Next you generate a random p value between 0.0 and 1.0 and walk through the cuimulative probabilities until the cumulative value exceeds p, and when that happens you return the index you’re at.
Suppose p = 0.47. Starting at index [0], the cumulative value in the next cell (0.10) is not greater than p = 0.47 so you advance. At index [1] the value in the next cell (0.35) is not greater than p so advance. At index [2] the value in the next cell (0.50) is greater than p so the result selected item is [2].
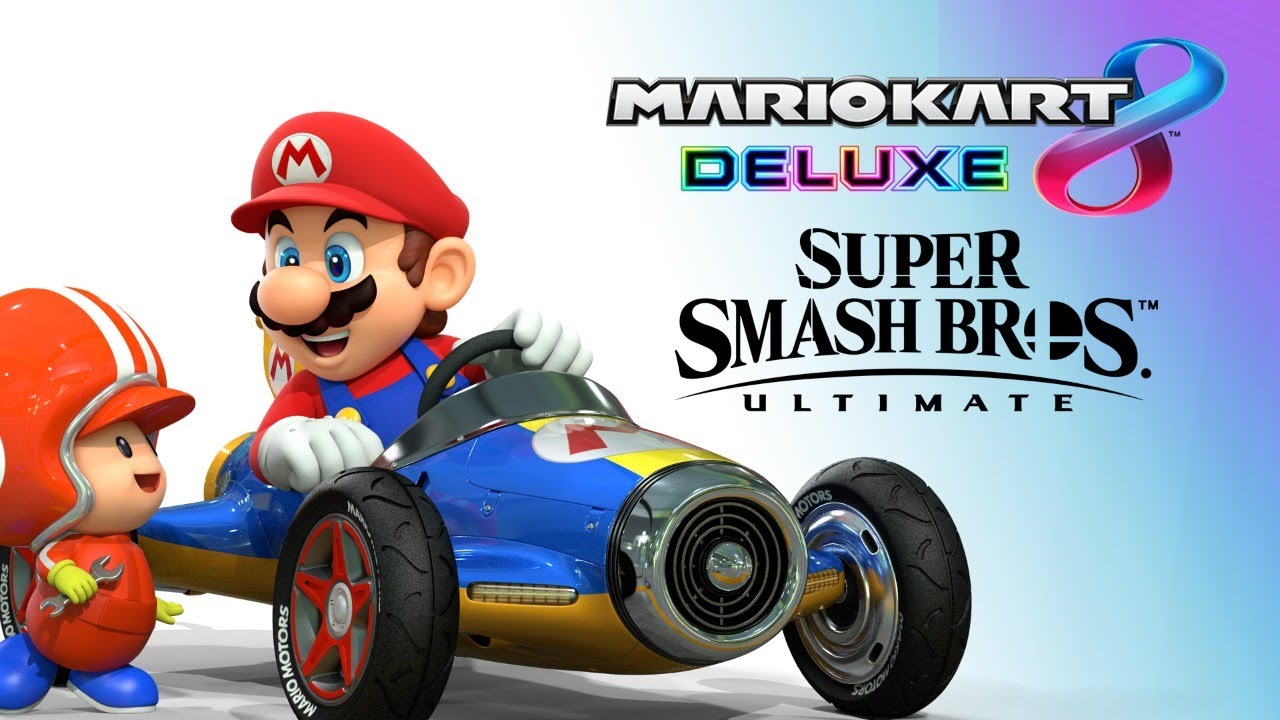
Here’s a C# implementation:
The implementation above is does unnecessary work in a sense. An alternative implementation computes probabilities and cumulative probabilities on the fly:
Computing on the fly will be slightly faster if you are called a proportinal selection function many hundreds of thousands of times, such as in a genetic algorithm.
To test my function(s), I wrote a little driver that calls the function on the demo data 1,000 times and compared the number of times each item was selected to the theoretical number of times.
Machine Learning Roulette App
Roulette wheel selection is so named because you can imagine a wheel made from the probabilities like so (for the demo data):
The roulette ball is thrown and will stop in one of the five slots but because the sizes of the slots is proportional to the data, slots that correspond to larger values have a higher probability of being landed in. Or something like that.
Three roulette wheel watches. Left: Jacob & Co. “Astronomia”- $620,000.00 — yes, you are reading this price correctly. Center: Invicta Model 28710 – $139.99. Right: Rolex Submariner – $12,900.00. The different prices of the watches are not proportional to their relative ability to tell time.
The results, which veer from the weird to the outright racist, draw attention to the bias of machine learning
The results, which veer from the weird to the outright racist, draw attention to the bias of machine learning
When I first saw ImageNet Roulette, I didn’t know what it was – only that an artist I follow on Twitter had posted an image of his face framed by a thin neon-green square, accompanied, in the top-left corner, by the words: ‘swot, grind, nerd, wonk, dweeb’. He then posted another photo, in which he had taken off his glasses; this time, the text in the frame read: ‘rape suspect’. And again, after having rearranged his hair, smiling: ‘first offender’.
Scrolling down, I started seeing those same green frames everywhere, tagged with words like ‘beard’, ‘mezzo-soprano, mezzo’, ‘cog’ and ‘weirdo’. It felt familiar: I’ve seen these kinds of tags before. Generated by ImageNet Roulette, a project by researcher Kate Crawford and artist Trevor Paglen, the labels draw on AI technology to replicate how machine learning systems analyze images. Using an open-source, deep-learning frame ImageNet Roulette matched uploaded images with categories that already exist in a training set called ImageNet. Such visual-recognition tests comprise some of the very first AI assignments, meaning there are numerous datasets of this kind. In their essay about the project, ‘Excavating AI: The Politics of Images in Machine Learning Training Sets’ (2019), Crawford and Paglen observe that they chose ImageNet – which was devised by computer scientists at Princeton and Stanford Universities between 2006 and 2009 – because it is the ‘canonical’ training set.
ImageNet Roulette allows users to upload a selfie to the site, where it is analyzed as it would be by AI. Although it does have a ‘people’ category, ImageNet is actually an object-oriented dataset that was never intended for facial-recognition training, and its tags for humans can be quite disturbing. ImageNet categorizes a human as ‘person, individual, someone, somebody, mortal, soul’ and then assigns to them one of thousands of labels, ranging from ‘journalist’ to ‘rape suspect’.
Video Roulette Machines In Casinos
Crawford uploaded to Twitter a photo of herself and Paglen, both wearing suits and looking very professional, in which she was tagged ‘newsreader, news reader’ and Paglen ‘microeconomist, microeconomic expert’. I tried it twice: initially, I was designated ‘nondriver’; then – following a slight change of scenery and position – ‘psycholinguist’, which appears to be a very common label. Another very common tag? ‘Face’. Some women posted on social media that they were flattered when ImageNet recognized them as ‘temptress’, ‘femme fatale’, ‘enchantress’ or ‘siren’. (Influencer Laura Lux uploaded a selfie with the comment ‘is ImageNet Roulette tryna fuck’ when her – surprisingly, not risqué – selfie got tagged ‘smasher, stunner, knockout, beauty, ravisher’.) One Twitter user uploaded the album cover of The Beatles’ Sgt. Pepper’s Lonely Hearts Club Band (1967), claiming that it ‘continues to be a great test of face neural networks’. ImageNet reads John, Paul and George (Ringo wasn’t detected) as ‘Fauve, fauvist’; there’s also a ‘cog’ and a ‘grinner’; someone in the corner is labelled ‘eager beaver, busy bee, live wire’; next to him is ‘policyholder’ and, close by, yet another ‘psycholinguist’.
The popularity of ImageNet Roulette on social media echoes that of FaceApp, which allowed users to change how old they looked in uploaded photos, and the Google Arts and Culture app, which paired users’ selfies with historical works of art. In the past couple of years, both these apps went viral, with users sharing the results across multiple social-media platforms. ImageNet Roulette’s aesthetic, with the distinct green frame and text, makes it even more appealing for sharing because it’s so instantly recognizable. Yet, while the three platforms operate similarly, they differ in intention. FaceApp and the Google Arts and Culture app collect data on users and are two worrisome examples of how viral participation distracts people who may otherwise be very conscious of their right to privacy. ImageNet Roulette does exactly the opposite: it draws users’ attention to systemic problems, in this case, the bias of a data set, which is exactly the kind of partiality that was exemplified by the Google Arts and Culture app, which has been widely criticized for racism, due to the inherent Eurocentrism of its data set that draws largely from Western museums, in which representations of people of colour are rare and, when they do appear, are largely historical documents of exploitation.
The language of ImageNet Roulette’s tags is so weird, and so removed from our experience (‘someone, somebody, mortal, soul’), that it’s hard to resist the impulse to share its results because they’re so alienated from reality and thus funny. (Or, like Alex Goldman, co-host of technology podcast Reply All, simply to have someone explain what the label ‘grass widower’ actually means.) The project reaffirms our notion – our hope – that machine thinking is still clunky, incapable. That computers are not superintelligent, uncontrollable beings; they may have billions of data points but they still can’t figure out language. We rejoice in the weirdness because, in the very moment that the machine strips us of our humanity by reading us as part of a dataset, it simultaneously reaffirms our humanity, since where it doesn’t see how its language is unresolved, we do – and we can laugh about it.
ImageNet Roulette reveals another issue with AI: judgement. In 2016, a group of scientists at Shanghai Jiao Tong University in China taught AI to recognize criminals by creating a training set with 1,000 faces of non-criminals and around 800 photographs of convicted criminals. The scientists discussed micro-expressions and countered those who claimed the idea was close to phrenology by observing that machines are neutral. Yet, as exemplified by Crawford and Paglen’s project – which forms part of their exhibition ‘Training Humans’ at Fondazione Prada in Milan – machines are only as unbiased as the training sets they are given to work with. Some of the ImageNet tags are so blatantly racist, misogynist, and hateful, that they are genuinely shocking, even in 2019. The telling-it-like-it-is mentality of the project feels offensive, but Crawford and Paglen explain in ‘Excavating AI’ that to see the foundation on which AI systems are trained is a ‘forensic method to understand how they work’ and continue – ‘this has serious consequences’.
Users’ delighting in the weirdness of being seen by a machine does not take away from their understanding of the ramifications of this vision. In a few days, or weeks, the internet will have forgotten about ImageNet Roulette, just as no one posts images from FaceApp anymore. Digital culture moves fast. Another, similar app will go viral soon, since users – humans, that is – are always curious about how they are seen. In an essay for the catalogue that accompanied ‘Astro Noise’, Laura Poitras’s 2016 exhibition at the Whitney Museum of American Art in New York, Crawford begins with a reference to the Oracle of the Temple of Apollo at Delphi, on which, legend has it, were once inscribed the words: ‘Know thyself.’
Main image: courtesy: ImageNet Roulette
Machine Learning Roulette Sites
MORE LIKE THISThe artist’s first feature film depicts a mother-daughter pair of grifters trying to stay afloat through financial recession
Jamila Prowse talks to three spaces - HOME, la Sala and Quench - about resisting cultural hegemony and the need for foundational support, access and equity in the arts
Shon Faye pays tribute to the pioneering musician and producer whose generation-defining music will live on in the exultations of the dance floor
Roulette Machines For Sale
Tributes to the late director of Wysing Art Centre, who supported a generation of artists in the UK